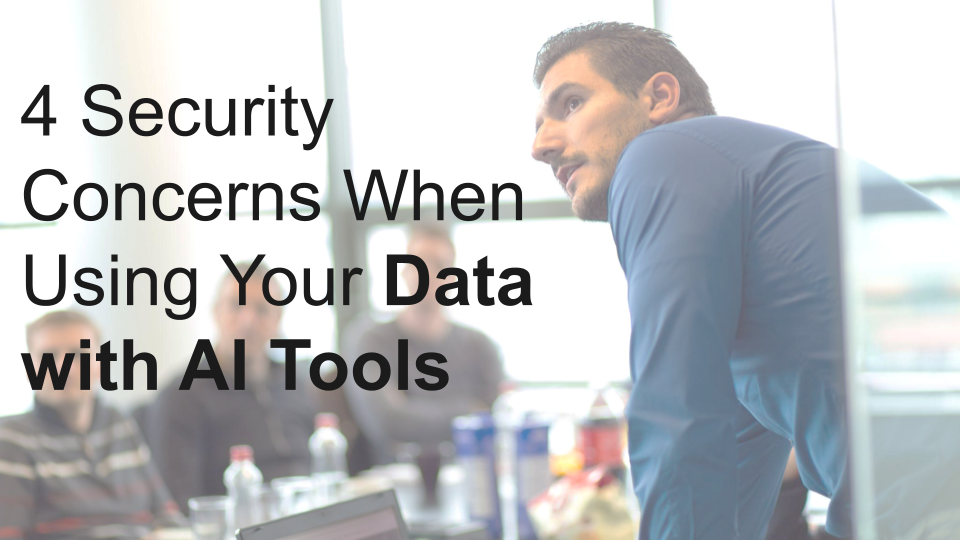
4 Security Concerns When Using Your Data with AI Tools
- Leyla Shokoohe
- July 26, 2024
- Cyber Security, JDE Security, Artificial Intelligence (AI)
- 0 Comments
Using AI has moved from a philosophical question to a practical one. The advantages of AI in the workplace are many: from executing simple tasks that free up employee time for bigger issues, routinely ordering of supplies; to creating a customer brief using your data, it’s no secret AI can be a big help. But not all AI tools are created equal. Some can leave you vulnerable to data breaches and security weaknesses. Even AI that is (seemingly) well vetted might still have loopholes your users aren’t considering.
That’s why it’s more important than ever to ensure your data remains secure when used with AI tools. Here are the top four security concerns we’re seeing when using AI with your private data, and things to consider before using these tools.
What’s the Big Deal with Data Security
Cybersecurity is your first line of defense for your data. If your proprietary data were to be exposed in a security breach and fall into the wrong hands, a lot could go wrong. AI tools work by scraping the whole of the internet and information available to them.
Say you’re a nonprofit with a fundraising wing. If one of your employees uses AI to create custom emails for donors, but plugs actual donor information into the AI, that action means that data becomes available for AI to scrape in the future, with no regard for privacy or sensitive information. In fact, broadly used AI GPTs, like ChatGPT and Gemini, have disclaimers that warn users that their data can and will be used elsewhere with no restrictions.
Companies will have varying internal cybersecurity policies in place. The most generic is the directive for employees to simply not use AI tools for work. As AI becomes more embedded in daily business transactions, this is less and less practical. Instead, companies need to direct users to avoid entering proprietary private data into public AI squares, as it were, like ChatGPT.
Problem #1: Unauthorized Use of AI Tools
This leads us to the first problem with AI and data security. Users are imperfect. User error can be common. The proliferation of readily accessible AI tools and their accepted use in the workplace has made user error even more likely. But when employees use AI content generators that their security team is unaware of, that’s where the worst danger lies.
Security officers may advise their companies to ban the use of content generators with employee work accounts, but employees may simply use their personal accounts instead. When this happens, they risk exposing sensitive, confidential and proprietary data to bad actors, because AI tools like ChatGPT save that data. Since public AI tools scrape the entirety of the internet for their content, that sensitive data, your organization’s lifeblood, becomes part of the AI repository.
As a possible solution, some companies may develop their own AI tools that are trained exclusively on their data and not accessible by outside users. However, say a user doesn’t like the results returned to them by their company GPT, and they throw those results into a public AI tool to be reworded or to get different ideas. All of a sudden, something that was private is now dumped into the public domain and once again exposed.
Problem #2: Risks of AI-Generated Code
Depending on your business needs and sector, code generation may fall within your employee/user purview. Or even if it doesn’t, your users may find themselves needing or wanting to generate code quickly and with low impact. If they turn to ChatGPT or another AI tool, they could end up building code that likely doesn’t meet security standards.
Another concern with generating code through ChatGPT is the source material the AI tool pulls from. If you’re not using a true development application, you are just accessing the same data anyone else can. ChatGPT returns results based on their massive Internet scraping – and there are likely threat actors who put out bad information. With no data discernment in place, you could be leveraging bad information and ingesting insecure practices that make it easier for bad actors to compromise your code.
Hackers can use the front end of the content generators to their advantage, too. Let’s use the code generating example. Nothing stops a hacker from posing the same queries or requests for code generation that the general public poses. But now, knowing that whatever these tools return is uniform across the board for all users for that specific query or request, they can use that broadly sourced information and reverse engineer it to figure out how they can breach it.
Problem #3: Potential Biases and Inaccuracies in AI Models
As the 21st Century adage goes, don’t believe everything you read…on the internet, especially. A plethora of inaccurate information exists on the internet, and when that information gets filtered through a user’s request in a GPT or other AI tool, there can be disastrous results.
Take for instance a lawyer. Say this lawyer needs to find more case law that supports their argument for their client. It’s best practice to consult tangible official materials and documents, but ChatGPT exists for moments like these, right? Why limit the supporting cases you can find to whatever is in the latest edition of a book or the most recent examples, when you could use ChatGPT or another AI tool to source relevant examples from across the entirety of the internet? Because, as some lawyers discovered in a 2023 aviation injury case, they might be sourcing fake examples and false information.
Other types of inaccuracies are more culturally taboo. In a widely published example in 2023, Gemini, Google’s AI, returned people of color to prompts for historical image generation. Many articles have been written about the racial bias problems that persist with images depicted through generative AI like Gemini or OpenAI. One example cited generated images of Black people in Nazi uniforms, which holds frightening implications regarding historical accuracy.
Problem #4: Decision Transparency
An increasing number of tasks are being outsourced to AI tools and various generative AI tools. When this happens, the nuances of human logic – and allowance for errors – are eliminated. But as we know, not everything, not even with AI, is black and white. AI that is trained to execute when certain scenarios occur could be forced to make a decision based on potentially false or misleading information, setting into motion a series of unintended events.
Take for example the case of Russia, China, and their nuclear capabilities. In May of 2024, senior U.S. officials called on these two countries to join the United States and other countries in declaring that any potential nuclear warfare decisions would be made by humans, not through AI algorithms. Should Russian radar incorrectly detect a threat which then triggers their AI into deploying a nuclear weapon, well, the repercussions of that false information are infinite.
Users need to ask themselves if they want AI unequivocally making decisions for them or not, especially based on the other three problems identified in this article. Best practice would insist on a system of checks and balances in place to have the final call come from a human.
Problem #5: Privacy Concerns, Compliance and Regulation
While privacy is at stake with all of these problems, it is also a problem in and of itself. Because the use of AI tools requires scraping the internet for relevant information, it is important to ensure that any information used is available to the public. Of course, it’s impossible to ensure that on the user end – if you input a prompt into Gemini, it’s very hard to trace the information to find out whether its sources gave their consent to the mass consumption of their personal data.
One step an organization can take is to require the complete pseudonymization or anonymization of any sensitive data available throughout an entire database. That way, if that database should be scraped, any data contained cannot be traced back to a source and reveal potentially private identification information.
Compliance and regulation also comes into play not only with the data available for scraping, but with the results of that scraping. As you may have noticed throughout this article, there are data concerns on both the front end and back end of AI tool usage for users.
The data that is returned is not filtered by AI to ensure it has met varying governmental regulations. These regulations can include everything from GDPR (General Data Protection Regulation, enacted in the EU but imitated by many American companies); HIPAA (Health Insurance Portability and Accountability Act) and the Gramm-Leach-Bliley Act in healthcare settings; BIPA (Biometric Information Privacy Act) for biometric data; and the FTC (Federal Trade Commission) and CCPA (California Consumer Privacy Act.)
Moving Forward, Informed
The onus of responsibility for data usage will ultimately fall on the user, short of any kind of regulation on AI tools. It is important for your users to be aware of the various problems that can arise with using data and AI tools. It is also important to have codified policies in place at your company as a first step to mitigating any kind of data safety and privacy issues.
Think it’s time to reexamine your company’s data security policy and plans? Our advisory team would love to speak with you about the possibilities. Drop us a line here.